AI in Finance: Enhancing Decision Making and Security
The finance sector, known for its complexity and dynamism, is experiencing a transformative shift with the integration of artificial intelligence (AI). From fraud detection to algorithmic trading and personalized customer services, AI is revolutionizing how financial institutions operate, make decisions, and secure transactions. In this blog post, we’ll explore the various ways AI is reshaping the financial landscape, its benefits, and the challenges it presents.
1. Fraud Detection and Prevention
Advanced Analytics and Real-Time Monitoring
AI has dramatically improved the accuracy and efficiency of fraud detection. Traditional methods often rely on rule-based systems, which can be circumvented by increasingly sophisticated fraud techniques. AI, on the other hand, utilizes advanced analytics and machine learning algorithms to identify unusual patterns and anomalies in real-time.
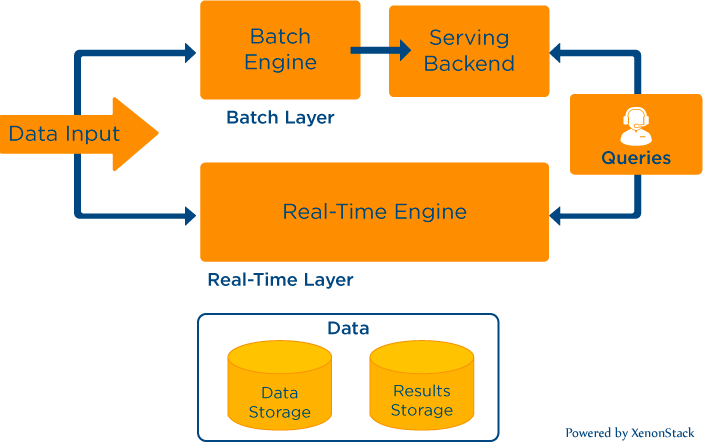
Real Time Analytics Definition. (2019). https://www.heavy.ai/technical-glossary/real-time-analytics
Machine Learning Models
These models continuously learn from historical data to predict and detect fraudulent activities. For instance, AI can analyze spending patterns on credit cards and flag suspicious transactions that deviate from the norm. The implementation of deep learning techniques has further enhanced the ability to detect even the most subtle fraudulent activities.
2. Algorithmic Trading and Investment Strategies
High-Frequency Trading
One of the most significant applications of AI in finance is algorithmic trading, where AI algorithms execute trades at high speeds and volumes. These systems analyze vast amounts of data, including historical prices, market conditions, and economic indicators, to make trading decisions in milliseconds. This high-frequency trading can capitalize on market inefficiencies that are undetectable to human traders.
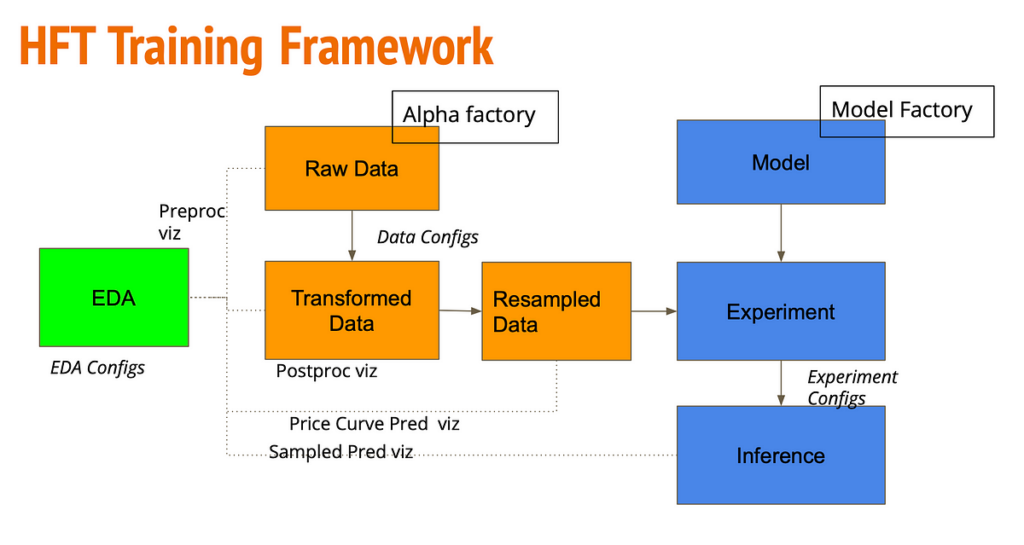
High Frequency Trading with AI: Towards Low-Risk, High-Profitability Trading. (2022, 18 de febrero). https://medium.com/quantland/high-frequency-trading-with-ai-towards-low-risk-high-profitability-trading-2a9568a9bb29
Predictive Analytics
AI-driven predictive analytics are also used to forecast stock prices, assess investment risks, and develop complex trading strategies. By leveraging machine learning models, investors can gain insights into market trends and make data-driven investment decisions. AI can also simulate various trading scenarios to optimize strategies and maximize returns.
3. Personalized Customer Service
AI Chatbots and Virtual Assistants
AI-powered chatbots and virtual assistants are transforming customer service in the finance industry. These tools provide instant, 24/7 support, handling inquiries ranging from account balances to loan applications. By understanding natural language and learning from interactions, they can offer personalized advice and solutions, enhancing the customer experience.
Financial Planning and Management
AI is also helping individuals and businesses manage their finances more effectively. Personal finance management apps use AI to analyze spending habits, predict future expenses, and offer budgeting advice. For wealth management, robo-advisors use AI to create and manage investment portfolios tailored to an individual’s financial goals and risk tolerance.
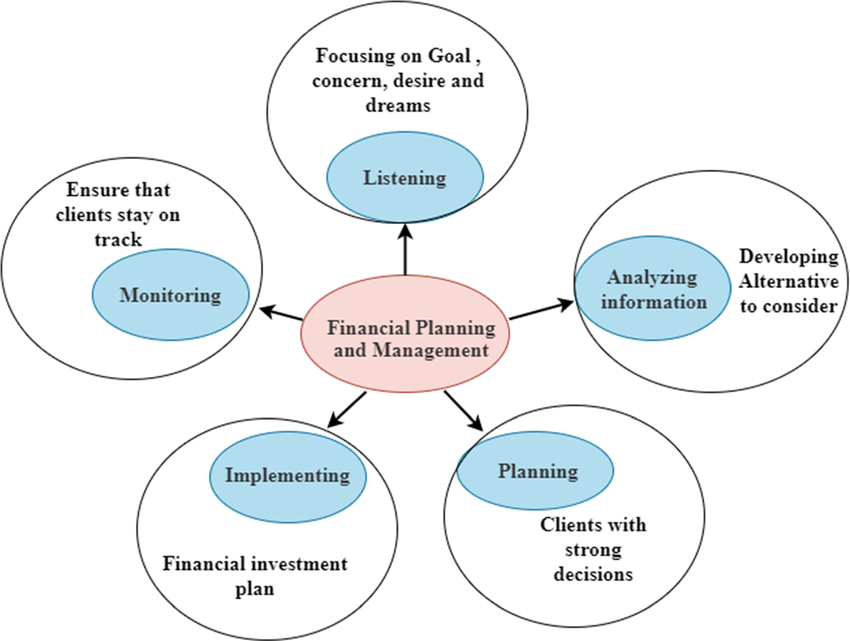
Lingyan, W., Mawenge, Rani, D. et al. RETRACTED ARTICLE: Study on relationship between personal financial planning and financial literacy to stimulate economic advancement. Ann Oper Res 326 (Suppl 1), 11 (2023). https://doi.org/10.1007/s10479-021-04278-8
4. Risk Management
Credit Scoring
Traditional credit scoring models often overlook subtle nuances in an individual’s financial behavior. AI-based credit scoring models consider a broader range of factors, such as transaction histories and social media activity, to assess creditworthiness more accurately. This holistic approach reduces the risk of default and broadens access to credit for individuals with limited credit histories.
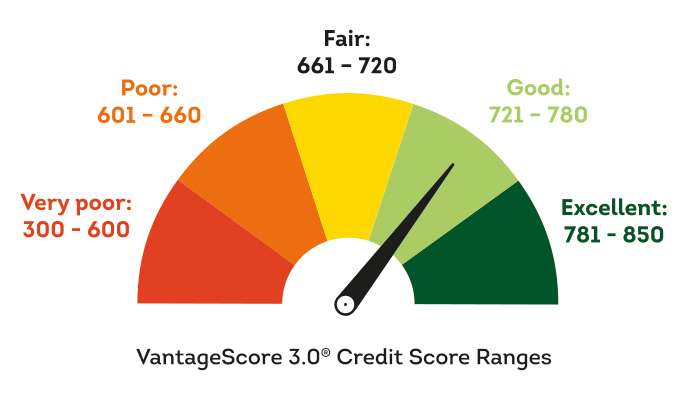
What is a good credit score?. (2022, 11 de febrero). https://www.transunion.com/blog/credit-advice/whats-considered-a-good-credit-score
Risk Assessment
AI is crucial in assessing and mitigating risks within financial institutions. By analyzing large datasets, AI can identify potential risks in loan portfolios, investment strategies, and operational processes. This proactive risk management enables institutions to take corrective actions before issues escalate.
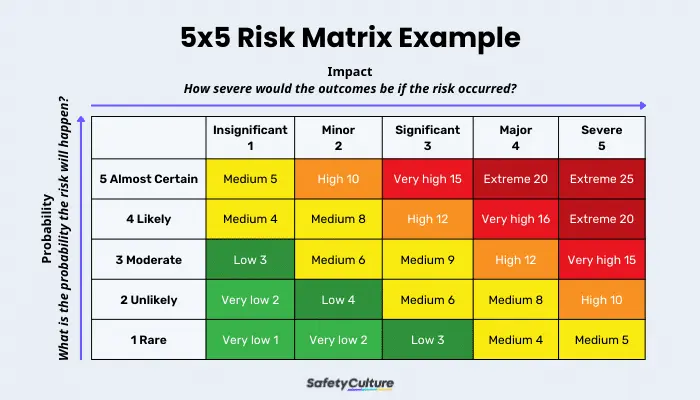
Patricia Guevara. (2024, 27 de marzo). A Guide to Understanding 5×5 Risk Assessment Matrix. https://safetyculture.com/topics/risk-assessment/5×5-risk-matrix/
5. Regulatory Compliance
Automating Compliance Processes
Financial institutions face stringent regulatory requirements that necessitate meticulous record-keeping and reporting. AI can automate these compliance processes, reducing the burden on human employees and minimizing the risk of errors. Natural language processing (NLP) algorithms can analyze legal documents, ensuring that financial practices adhere to regulatory standards.
Anti-Money Laundering (AML)
AI is instrumental in combating money laundering by identifying and flagging suspicious transactions. Machine learning models analyze transaction data, customer profiles, and behavioral patterns to detect and report activities that may indicate money laundering, thus enhancing regulatory compliance.

Ian Correa. (2022, 18 de marzo). How to Clean Up Your Data for Anti-Money Laundering (AML) Compliance. https://www.precisely.com/blog/data-quality/clean-data-anti-money-laundering-compliance-aml
Challenges and Ethical Considerations
Data Privacy
With AI’s reliance on vast amounts of data, ensuring the privacy and security of sensitive financial information is paramount. Financial institutions must implement robust data protection measures and comply with data privacy regulations to prevent breaches and misuse of information.
Bias and Fairness
AI systems can inadvertently perpetuate biases present in the training data, leading to unfair outcomes in credit scoring, loan approvals, and other financial decisions. It is crucial to develop and deploy AI models transparently, regularly auditing them for bias and ensuring fairness in their applications.
Regulatory Landscape
As AI continues to evolve, so does the regulatory landscape. Financial institutions must stay abreast of changing regulations and ensure their AI systems comply with legal standards. Collaborative efforts between regulators, industry leaders, and AI developers are necessary to create frameworks that balance innovation with consumer protection.
Conclusion
AI is undeniably transforming the finance industry, offering enhanced decision-making capabilities, improved security, and personalized customer experiences. While the benefits are substantial, it is essential to address the associated challenges and ethical considerations. As AI technology continues to advance, its role in finance will likely expand, paving the way for a more efficient, secure, and inclusive financial ecosystem. Financial institutions that embrace AI’s potential and navigate its challenges will be well-positioned to thrive in the evolving digital landscape.